Â
We are thrilled to extend an invitation to you for a seminar series, where we will unravel the latest breakthroughs in anomaly detection using cutting-edge machine learning approaches.
đ Event Details:
- Event Name: Anomaly Detection using Artificial Intelligence
- Date: 18th – 22nd March 2024
- Time: 10:00 – 13:00 CET (Mon, Tue, Thu, Fri)
- Venue: Online (via MS Teams)
The seminar is a unique opportunity to connect with experts and consortium members within the European Project community. We will not only showcase our expertise but also present an enlightening case study that explores the intricacies of anomaly detection and its pivotal role in safety-critical systems.
Join us as we share insights into our methodologies, delve into the challenges faced, and unveil the impactful outcomes achieved. This event is designed to foster collaboration, encourage knowledge exchange, and strengthen ties within our dynamic community.
đ Why Attend?
Gain in-depth knowledge of advanced anomaly detection techniques.
Explore the implications of machine learning in safety-critical systems.
Network with professionals and researchers in the European Project community.
đ§ RSVP and contact information
To confirm your attendance or for further details, please register your interest at this form or book a free spot via Eventbrite.
Your participation is not just an acknowledgment of our progress; it’s an opportunity to collectively shape the future of collaborative intelligence in safety-critical systems.
We look forward to your presence at this momentous event!
Course schedule
Scope of the seminar:
Types of anomalies:
- Pattern
- Point
- Drift
Types of data:
- Time-series
- Image data
- Tabular data
Time-Series:
- Feature Engineering
- Univariate – multivariate
- Feature representation and encoding (sliding window)
- Models with state and history
- RNNs and LSTMs
Point:
- Classifications (class imbalance, f1 score):
Types of Metrics:
- Precision
- Recall
- F1 Score
- Confusion Matrix
- MSE (Mean Squared Error)
- AIC (Akaike Information Criterion)
- BIC (Bayesian Information Criterion)
- Kullback-Leibler Divergence
- Sensitivity
- Jaccard Index
Types of Methods:
Deep learning for anomaly detection
- Classical Change Point Detection (Scenarios and Methods)
- Some Online Anomaly Detection Scenarios
- Sequential Analysis (Sequential Tests and Control Charts)
- Comparison of Performances
- Modern Predictive Modeling
- Probabilistic Techniques
- BFAST
- Extended CUSUM
- Explainable Change Point Detection
- Explainable AI Techniques for Time Series
- Local Surrogate Modelling for CPD
Bayesian network for anomaly detection:
- Using uncertainty to detect Anomalies
- Using user-defined thresholds to classify anomalies
Case studies in safety-critical industries:
- Industrial maintenance
- Automotive industry
- Chemical process plants control
- RoboticsÂ
About the speakers:
Prof. John Kelleher
Prof. Kelleher has over two decades of research experience in artificial intelligence, a field that has witnessed significant transformation since the emergence of generative AI. His research innovations have shown impressive abilities in a range of domains, from healthcare to the sustainability of AI models.
Prof. Kelleher’s research interests focus on harnessing AI to enhance the understanding and treatment of complex medical conditions. For example, his work in stroke care is pioneering, utilising AI and digital twins in the Stratif-AI project to revolutionise risk assessment and enhance prevention, treatment, and rehabilitation throughout a personâs life.
Additionally, through the EU-funded Validate project, he is developing a prognostic tool to predict outcomes for acute stroke patients, translating AI research into vital clinical tools. And leading the data science efforts in RES-Q+, he contributes to a global initiative aimed at elevating stroke care quality, showcasing his commitment to impactful healthcare improvements through AI.
Prof. Kelleher also does a significant amount of research in natural language processing, including work on large-language models and machine translation, with a particular focus on improving the energy and data efficiency of these systems to make them more sustainable and applicable in low-resource contexts.
He is the author of multiple books that have been widely praised for their intellectual rigour and accessibility and has published over 200 peer-reviewed papers in the fields of AI and machine learning in the last five years alone. In addition, he has a history of successful collaboration with industry partners and has secured over âŹ11 million in competitive research funding from a variety of sources.
Prof. Kelleher has excelled as an academic leader and educator, demonstrated in part through his role as one of the co-Principal Investigators and as institutional lead in the SFI Centre for Research Training in Digitally Enhanced Reality (D-REAL), training 120 PhD students across five partner universities. He has won international praise for his ability to make his scholarship accessible to the wider public. As such, he is considered one of Irelandâs most gifted communicators in the area of AI and machine learning.
Dr. Florian Sobieczky
Dr. Florian Sobieczky is a senior researcher in the Data Science Lab at the Software Competence Center Hagenberg focussing on Machine Learning for Fault Detection. After obtaining his diploma in physics, Florian Sobieczky earned his doctorate in Mathematics at the University of Göttingen with a thesis about percolation theory. During his dissertation, he worked as an assistant at Berlin Technical University in teaching and research in the field of mathematical physics. As a post-doc Florian Sobieczky worked at the Mathematics Department (Institut C) of Grazâ University of Technology, at the ‘Mathematisches Insitut’ of the University of Jena, and as a guest-professor at the University of Colorado at Boulder (CU). From 2012 until 2015 he was a lecturer in Mathematics at the University of Denver (DU). In 2016 he joined the Software Competence Center Hagenberg.
Florian Sobieczkys research is in probability theory, particularly discrete stochastic processes. He has published in internationally renowned journals in the fields of percolation theory, random walks on graphs, and queueing theory, and organized two workshops at international conferences with proceedings published with BirkhÀuser and the American Mathematical Society. The projects at SCCH to which he is typically contributing are related to the statistics of production processes. Fault detection, anomaly diagnosis and predictive maintenance are key tasks for which his skills find applicability.
As a senior scientist at SCCH, Florian Sobieczky is devoted to the reseach associated with fault detection and prescriptive maintenance in industrial production processes. The conventional application of predictive machine learning models to production data is usually enhanced if “third party” knowledge (e.g. in the form of stochastic or physical models) is included into the analysis. The typical goal is to make high performing prediction algorithms work hand in hand with interpretable classical models, thus rendering the increase of predictive performance interpretable in the sense of the underlying physical or stochastic model. In the context of Explainable Artificial Intelligence (XAI) Florian Sobieczky is involved in the project “inAIco” (Interpretable Artificial Intelligence Corrections), a BRIDGE program of the Austrian Industrial Science Foundation (FFG), which has been granted in April 2020. It is a three-year project with the aim of delivering explanations of the predictions of high performing machine learning methods for which AI acts as a “corrector” on top of the predictions of the classical model.
Dr. Florian Kromp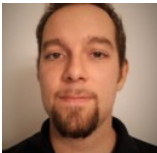
Florian currently serves as a Senior Researcher at Software Competence Center Hagenberg. With a commitment to advancements in healthcare, Florian has held key roles at St. Anna Kinderspital, contributing as an IT-Berater and IT-Experte. He also worked at the Children’s Cancer Research Institute as a Bioimage Informatician. Florian has earned his Ph.D. in Computer Science from Technische UniversitĂ€t Wien, following completion of his Diplom-Ingenieur in Medical Informatics.
Johannes Zellinger   Â
Â
Johannes is a Researcher and Data Scientist at Software Competence Center Hagenberg GmbH (SCCH) in Weibern, Upper Austria, Johannes brings over two years of invaluable experience to his role. Before his tenure at SCCH, Johannes worked as a Contractor at NXP Semiconductors for five years. Johannes holds a Master’s degree in Information and Computer Engineering from Technische UniversitĂ€t Graz, where he also pursued studies in Biomedical Engineering. His academic journey began at Linzer Technikum HTL Paul-Hahn-StraĂe, where he studied Electrical Engineering and Automation.
Lamia Zain 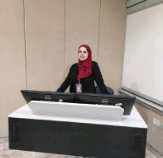
Lamia is currently pursuing a Master’s Degree in Mechatronics at Nile University, Egypt. Lamia has earned certifications in Introduction to SQL, EON-XR Educator, and has completed the Artificial Intelligence Nanodegree. Currently, she is serving as an Assistant Lecturer at The Knowledge Hub University and a Session Lead at Udacity. She has also served as a Tutor at the Global Academic Foundation and as a Teaching Assistant at Nile University for over four years. Lamia has contributed to the advancement of education as a Certified Teacher at the Information Technology Institute (ITI) in Cairo, completing the AI-Pro 9-month program. Lamia’s academic journey began at Benha University, where she earned her Bachelor’s degree in Mechatronics, Robotics, and Automation Engineering.
About the instructors:
Aayush Jain 
Aayush pursued his bachelorâs studies at VIT University (India), obtaining a degree in Mechanical Engineering with a thesis investigating methods for intelligent precision machining operations using serial manipulators. His passion for robotics and applied AI emerged during the course of his undergraduate studies. To further the experience, he spent two semesters at Advanced Intelligent Robots Lab in Taiwan as a Visiting Researcher. Later, He was awarded a fully funded Professors Scholarship to pursue masterâs studies at the National Taiwan University of Science and Technology. His work in Taiwan introduced a novel model-based intelligent force/position compliance end-effector aimed to develop inexpensive precision machining technologies to further enable Industry 4.0 in SMEs. Many of his works are driven by his search for âintelligentâ and âpracticalâ solutions to modern-day problems. Currently, he is pursuing a Ph.D. in Imitation Learning for Safe Human-Robot Collaboration under the Marie SkĆodowska-Curie fellowship program (Ireland, Italy, Serbia). In this project, heâll be investigating how exteroceptive sensors, human-in-the-loop control architecture, and learning from demonstration can improve the productivity of a cobot cell. His aim is to develop a framework for learning human behaviors and skills through demonstration to cut down the task definition time for collaborative flexible assembly lines.
Devesh Jawla 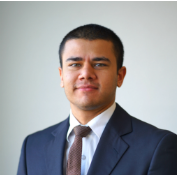
His PhD research centers on the topic of Active Learning for fault detection. Despite significant progress in Artificial Intelligence research in recent years, practical applications often rely on simple models. While supervised learning enables tasks like classification and regression, the performance of these models is constrained by the availability of labeled data. The current challenge is not data scarcity but the need for labeled data, and obtaining this data requires human annotators, often spending countless hours on the task. The central research question that he addresses is how to design intelligent algorithms capable of reducing the annotation cost. The proposed solution lies in Active Learning, where an algorithm serves as a counterpart to him, the human annotator. This algorithm actively seeks and learns the most valuable information, aiming to achieve performance comparable to a fully labeled dataset.
Ammar Abbas 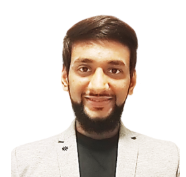
Ammar has a master’s degree in Mechatronics through the Erasmus Mundus program (Egypt, France, & Spain) and currently pursuing a Ph.D. in Deep Reinforcement Learning under the Marie SkĆodowska-Curie fellowship program (Austria, Ireland, Italy). His objective is to improve the deficiencies of society with technology. He has done his bachelorâs in Mechanical engineering from Pakistan and his research interest starting from Mechatronic systems and structures converges to Robotics and Artificial Intelligence (AI). He has several experiences under different startups related to STEM education and robotics companies. Further, innovative Green Energy Technology is his another field of interest. In this project, he is focusing on the optimal decision-making process in safety-critical systems such as industries (detecting anomalies and prescriptive maintenance, optimizing the quality of the product, process scheduling, etc) or in the human-robot collaborative environment. The idea is to use the “Reinforcement Learning approach as an online learning method”, considering human-in-the-loop setting by combining the shared mental models of human experts or operators with the data-driven explainable and interpretable AI.
Joseph Mietkiewicz 
Joseph is studying Bayesian Network to help decision-making in collaboration with Hugin Expert A/S company. About his Research at CISC he says In modern industry, Human operators are crucial for the well-being of plants. The operator has an indication of deviance in the process thank to alarms. But with the increasing complexity of the modern industry, the number of alarms skyrocketed. The operator can be confronted with a high number of alarms that can be misleading. The idea of this project is to develop Bayesian Network models that help decision-making for a human operator in case of a process upset. Bayesian network is a machine learning technique that can be used to extract useful alarms from an alarm flow and predict critical events. I am currently working on building a data-driven Bayesian network that predicts critical events and indicates which alarms are the most important related to this critical event.
Module Descriptions
Prof. John Kelleher:
Time Series Overview
Feature Engineering in Time-series
RNNs
Dr. Florian Sobieczky:
- Classical Change Point Detection (Scenarios and Methods)
- Online Anomaly Detection Scenarios
- Sequential Analysis (Sequential Tests and Control Charts)
- Comparison of Performances
- Modern Predictive Modeling
a. Probabilistic Techniques
b. BFAST
c. Extended CUSUM
6. Explainable Change Point Detection
a. Explainable AI Techniques for Time Series
b. Local Surrogate Modelling for CPD
Dr. Florian Kromp and Johannes Zellinger:
- Visual anomaly detection (AD) methods
a. Overview of visual AD approaches
b. Metrics for evaluation
2. Case study: robustness of one-class classification
a. Challenges in productive environments
b. Sample efficiency of one-class classification (negative supervised learning) strategies
c. Robustness of state-of-the-art one-class AD methods
I. Patch-based approaches
II. Student-teacher approaches
III. Diffusion Model based approaches
Lamia Zain:
Anomaly detection recap and in a nutshell
Case study: using a machine learning model to monitor the temperature of some system.
Aayush Jain:
Anomaly detection overview
Types of anomalies
Types of data
Anomaly detection in robotics
Ammar Abbas:
Types of metrics
Types of methods
Anomaly detection using HMM Maintenance Turbofan Process control Control room Robotics
Devesh Jawla:
Anomaly Detection for tabular and image datasets using Bayesian Neural Networks
- Using uncertainty to detect Anomalies
- Using user-defined thresholds to classify anomalies
Â